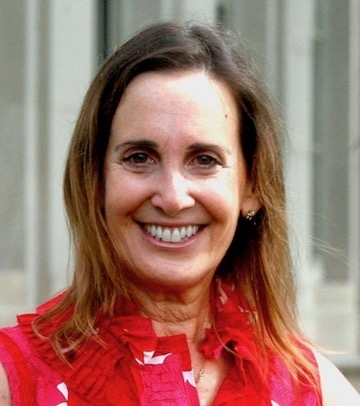
Bonnie Berger, Professor of Applied Mathematics and Computer Science. Among the first algorithmic computer scientists to enter the field of computational molecular biology, making fundamental contributions to structural bioinformatics, viral shell assembly and mis-assembly, comparative genomics, protein networks, and theoretical models of protein folding. Played a major role in shaping these fields as a mentor to young researchers. Influenced many subareas of computational biology, largely via algorithmic insights; introduced probabilistic modeling to protein fold recognition; founded and developed conservation-based methods for comparative genomics; and solved a difficult theoretical problem central to the biophysics and protein folding communities. Showed how to globally align protein interaction networks using an eigenvalue formulation, and used these alignments to uncover functionally related proteins across model organisms. Recently invented “compressive genomics” and demonstrated that algorithms that compute directly on compressed data allow the analysis of genomic data sets to keep pace with data generation. Close collaborations with experimental biologists have guided her computational work and given it significant impact in the laboratory. Hundreds of biology labs rely on software she developed and made freely available. Member, American Academy of Arts and Sciences. Fellow, International Society for Computational Biology. Fellow, Association for Computing Machinery. Named one of Technology Review’s inaugural 100 Young Innovators of 1999. In 2011, selected to deliver the Margaret Pittman Director’s Lecture at the National Institutes of Health.
RECOMB 2015 keynote talk: Computational biology in the 21st century: Algorithms that scale
The last two decades have seen an exponential increase in genomic and biomedical data, which will soon outstrip advances in computing power. Extracting new science from these massive datasets will require not only faster computers; it will require algorithms that scale sub-linearly in the size of the datasets. We show how a novel class of algorithms that scale with the entropy of the dataset by exploiting its fractal dimension can be used to address large-scale challenges in genomic search, sequencing and small molecule search.